aiida-core version: 1.0.1
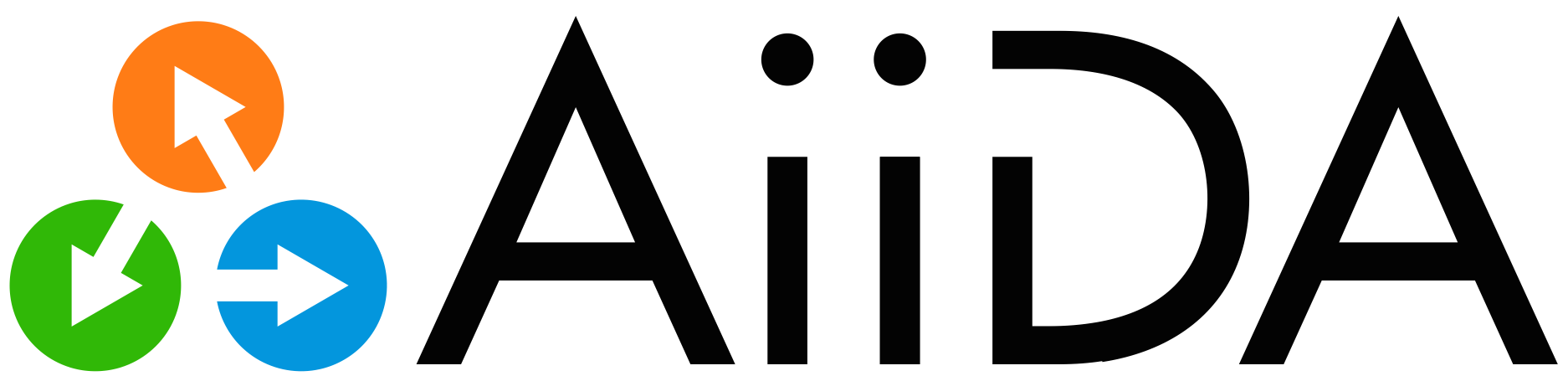
Fig. 1 Automated Interactive Infrastructure and Database for Computational Science¶
Welcome to AiiDA’s documentation!¶
AiiDA is a python framework that aims to help researchers with managing complex workflows and making them fully reproducible.
Features¶
Workflows: Write complex, auto-documenting workflows in python, linked to arbitrary executables on local and remote computers. The event-based workflow engine supports tens of thousands of processes per hour with full checkpointing.
Data provenance: Automatically track inputs, outpus & metadata of all calculations in a provenance graph for full reproducibility. Perform fast queries on graphs containing millions of nodes.
HPC interface: Move your calculations to a different computer by changing one line of code. AiiDA is compatible with schedulers like SLURM, PBS Pro, torque, SGE or LSF out of the box.
Plugin interface: Extend AiiDA with plugins for new simulation codes (input generation & parsing), data types, schedulers, transport modes and more.
Open Science: Export subsets of your provenance graph and share them with peers or make them available online for everyone on the Materials Cloud.
Open source: AiiDA is released under the MIT open source license.
See also the AiiDA home page.
Getting started¶
The AiiDA tutorials are a good place to get started with using AiiDA.
Afterwards, you might want to install AiiDA on your machine.
For setting up a production environment, you may find the configuration section helpful.
For the advanced, there is the complete AiiDA API reference (including a Module Index), and of course you can always peek into the code on the AiiDA git repository.
For developers¶
If you wish to start developing a plugin for AiiDA the Basics section is a good place to start.
Practical information concerning how to set up your development environment and how to contribute to aiida core can be found on the AiiDA Wiki.
You are also more than welcome to join the AiiDA mailing list or contribute to aiida-core on GitHub.
How to cite¶
If you use AiiDA for your research, please cite the following work:
Giovanni Pizzi, Andrea Cepellotti, Riccardo Sabatini, Nicola Marzari, and Boris Kozinsky, AiiDA: automated interactive infrastructure and database for computational science, Comp. Mat. Sci 111, 218-230 (2016); https://doi.org/10.1016/j.commatsci.2015.09.013; http://www.aiida.net.
Acknowledgements¶
This work is supported by the MARVEL National Centre for Competency in Research funded by the Swiss National Science Foundation, as well as by the MaX European Centre of Excellence funded by the Horizon 2020 EINFRA-5 program, Grant No. 676598.
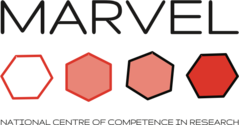
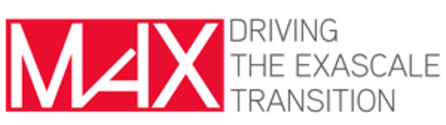